The Role of AI in Hedge Funds and Private Equity
Introduction
The world of finance is constantly evolving, and one of the most transformative forces in recent years has been artificial intelligence (AI). AI is not just a buzzword; it’s a powerful tool that has revolutionized various aspects of the financial industry. In this blog post, we will delve into the intriguing world of AI’s influence on hedge funds and private equity, two investment vehicles that have traditionally relied on expert human judgment and intricate financial analysis.
Understanding Hedge Funds and Private Equity
Before we explore AI’s impact, let’s begin by understanding what hedge funds and private equity are and how they differ from traditional investment vehicles.
Hedge Funds: Hedge funds are investment funds that pool capital from accredited individuals and institutional investors to invest in a diverse range of assets. These assets can include stocks, bonds, currencies, commodities, and derivatives. Hedge funds are typically known for their aggressive investment strategies and aim to provide investors with high returns.
Private Equity: Private equity, on the other hand, involves investing in privately held companies, either by acquiring them outright or taking substantial ownership stakes. Private equity firms work to improve the performance of these companies, often with a focus on long-term growth. Private equity investments are typically illiquid, with a longer investment horizon.
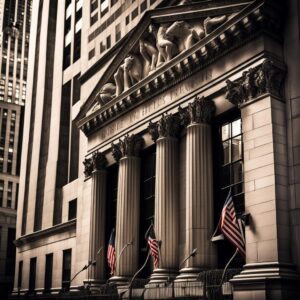
Historical Context
Both hedge funds and private equity have a rich history dating back several decades. Hedge funds emerged in the mid-20th century, initially designed to “hedge” against market downturns. Over time, they expanded their strategies and became known for their innovative and, at times, risky approaches to investing.
Private equity, on the other hand, gained prominence in the 1980s with leveraged buyouts and became synonymous with transforming and revitalizing struggling companies. Both of these investment avenues have played a pivotal role in shaping the financial landscape.
The Evolving Landscape of Asset Management
In recent years, the asset management landscape has evolved significantly. The traditional approach, which relied heavily on human expertise and analysis, is being complemented and, in some cases, supplanted by AI-driven solutions.
Traditional vs. AI-Powered Asset Management: Traditional asset management often involved extensive research, fundamental analysis, and an understanding of market psychology. However, AI-powered asset management adds a new dimension by leveraging massive datasets, sophisticated algorithms, and machine learning to make data-driven investment decisions. This shift from human intuition to data-driven decision-making is a defining feature of AI’s role in finance.
The Need for Data-Driven Decision-Making: One of the key driving forces behind the adoption of AI in finance is the sheer volume of data available. Financial markets generate an enormous amount of data every second, and AI has the ability to process and analyze this data at an unprecedented scale. This data-driven approach enables investment professionals to make more informed and timely decisions.
The Role of Technology in the Financial Industry: AI’s influence extends beyond hedge funds and private equity; it’s transforming the entire financial industry. In banking, insurance, and even personal finance, AI is enhancing customer experiences, automating processes, and improving risk management. It’s no longer a question of whether AI will play a role; it’s a matter of how significant that role will be.
AI in Investment Decision-Making
Now that we’ve explored the changing landscape of asset management, let’s delve deeper into how AI is used in investment decision-making within hedge funds and private equity.
How AI is Used in Investment Analysis: AI’s application in investment analysis is multifaceted. It involves a combination of data collection, data processing, predictive analytics, and modeling. Let’s break down these elements:
- Data Collection and Processing:
- AI algorithms can collect and process vast amounts of structured and unstructured data from various sources. This includes financial reports, news articles, social media, and even satellite imagery. The ability to assimilate and process this data is a game-changer.
- Predictive Analytics and Modeling:
- AI models use historical and real-time data to make predictions and identify trends. For example, machine learning algorithms can analyze stock price movements and identify patterns that are difficult for humans to discern. These insights can inform investment decisions.
Machine Learning and its Applications: Machine learning is a subset of AI that plays a central role in financial analysis. Here are a few ways in which machine learning is applied:
- Pattern Recognition and Trend Analysis:
- Machine learning models excel at recognizing patterns in data. In financial markets, this translates to identifying trends, correlations, and anomalies that can inform trading decisions.
- Risk Assessment and Mitigation:
- Machine learning models are used to assess and mitigate risks in investment portfolios. By analyzing historical market data and potential risk factors, AI can help create more resilient investment strategies.
Benefits of AI in Hedge Funds and Private Equity
The integration of AI into hedge funds and private equity brings a multitude of benefits. Let’s explore some of these advantages:
Improved Decision Accuracy and Consistency: AI systems can make investment decisions with a level of accuracy and consistency that’s challenging for humans to achieve. These systems are not influenced by emotions or biases and can process information in real-time.
Enhanced Risk Management: One of the key functions of AI in finance is risk management. By continuously monitoring and assessing risk factors, AI can help in the early identification of potential risks and the development of strategies to mitigate them.
Identifying Untapped Investment Opportunities: AI can process vast datasets to identify investment opportunities that might be hidden from human analysts. It can analyze data from different sources and generate insights that human analysts may overlook.
Reducing Human Bias and Emotions in Decision-Making: Human emotions and biases can cloud judgment and lead to suboptimal investment decisions. AI systems, being devoid of emotions, can make rational decisions based on data and predefined rules.
Cost-Efficiency and Scalability: AI systems can significantly reduce operational costs in the long run. Once developed and deployed, AI algorithms can handle a high volume of tasks without a proportional increase in cost.
AI in Quantitative Strategies
Within the world of hedge funds, quantitative strategies have gained prominence, and AI has become a crucial component in implementing these strategies.
Overview of Quantitative Hedge Funds: Quantitative hedge funds, often referred to as “quants,” use mathematical and statistical models to make investment decisions. These funds rely on quantitative analysis, data-driven models, and algorithms to identify profitable trading opportunities.
How AI is Employed in Quantitative Trading Strategies: AI plays a pivotal role in enhancing the capabilities of quantitative hedge funds. Here are a couple of ways in which AI is integrated into quantitative strategies:
High-Frequency Trading:
- AI algorithms are used to execute high-frequency trading strategies. These algorithms can analyze market data and execute trades within microseconds, capitalizing on fleeting market opportunities.
Statistical Arbitrage:
- Statistical arbitrage involves identifying price disparities between related assets and executing trades to capture profit from these price differentials. AI is used to analyze historical data and identify patterns that suggest future price movements.
Real-World Examples of AI-Driven Quantitative Funds’ Success: Quantitative hedge funds employing AI strategies have achieved notable success. Renaissance Technologies, a prominent quant fund, has demonstrated the power of AI in finance. Their Medallion Fund, which primarily relies on AI-driven strategies, has consistently delivered exceptional returns.
AI in Private Equity Deal Sourcing
In the realm of private equity, AI is being used to streamline the often complex process of deal sourcing.
Challenges in Private Equity Deal Sourcing: Private equity deal sourcing involves identifying and evaluating potential investment opportunities. This process can be labor-intensive and time-consuming, often requiring significant human effort to discover suitable targets.
How AI Streamlines Deal Origination and Due Diligence: AI solutions have emerged to simplify deal sourcing in private equity. Here’s how AI is contributing:
- Automated Data Analysis:
- AI can analyze vast amounts of data to identify potential investment targets. It can scan financial reports, news articles, and other data sources to flag companies that meet specific criteria.
- Natural Language Processing (NLP):
- NLP algorithms can process and understand text-based data, making it easier to extract valuable information from unstructured sources, such as news articles and social media.
Success Stories of AI-Powered Private Equity Firms: Private equity firms have embraced AI in their deal sourcing and due diligence processes. By automating the initial stages of target identification and evaluation, they can focus their human expertise on the more critical aspects of deal making.
Ethical Considerations in AI-Driven Finance
While AI brings about numerous advantages in finance, it also raises ethical concerns that need to be addressed.
Addressing Concerns of Transparency and Fairness: AI algorithms can sometimes operate as “black boxes,” making it challenging to understand the rationale behind their decisions. Ensuring transparency and fairness is a critical ethical consideration.
The Role of Regulations and Compliance: Financial regulators are working to develop guidelines and regulations that address the ethical use of AI in finance. Compliance with these regulations is crucial to maintain the trust of investors and the public.
The Human Touch in AI-Augmented Finance: AI should be seen as a tool that complements human expertise rather than replacing it. The most successful firms are those that find the right balance between AI and human decision-making.
Risks and Challenges
As with any technological advancement, AI in finance comes with its share of risks and challenges.
Data Quality and Privacy Concerns: AI systems are only as good as the data they are trained on. Ensuring the quality and accuracy of data is paramount to making informed decisions. Additionally, data privacy concerns are ever-present, particularly when handling sensitive financial information.
Vulnerabilities to Market Shifts and Black Swan Events: AI models are trained on historical data, which may not account for unprecedented events. Black swan events, such as the global financial crisis in 2008 or the COVID-19 pandemic, can expose vulnerabilities in AI-driven strategies.
Ethical Dilemmas in Algorithmic Decision-Making: AI systems can inadvertently perpetuate biases present in historical data. This can lead to discriminatory outcomes and ethical dilemmas that must be addressed by developers and users of AI technology.
Case Studies
To illustrate the real-world impact of AI in hedge funds and private equity, let’s examine a few case studies.
- Renaissance Technologies and the Medallion Fund:
- Renaissance Technologies, founded by James Simons, has achieved exceptional returns using AI-driven quantitative strategies. The Medallion Fund, which is primarily AI-powered, has consistently outperformed market benchmarks.
- The Carlyle Group’s Investment in Veritas:
- The Carlyle Group, a global private equity firm, used AI and data analytics to inform its investment in Veritas Technologies. The technology and data-driven approach enabled them to make an informed decision about the company’s potential for growth and value creation.
The Future of AI in Hedge Funds and Private Equity
AI’s role in hedge funds and private equity is not static; it continues to evolve. Let’s explore what the future holds for AI in these investment vehicles.
Trends and Emerging Technologies in AI for Finance: Several trends are shaping the future of AI in finance:
- Explainable AI: Developing AI models that provide explanations for their decisions is a growing area of research. This can enhance transparency and trust in AI-driven systems.
- Quantum Computing: Quantum computing holds the potential to revolutionize financial modeling and simulations, enabling faster and more accurate calculations.
- Blockchain and Smart Contracts: These technologies have the potential to streamline and automate aspects of financial transactions, reducing the need for intermediaries.
Anticipated Developments and Challenges: As AI in finance continues to advance, challenges and opportunities will arise. Some developments to anticipate include:
- Enhanced Risk Assessment: AI models will become even more sophisticated in assessing and mitigating risks, potentially leading to more resilient investment strategies.
- Regulatory Advancements: Regulatory bodies will continue to adapt to the evolving AI landscape, providing clearer guidelines for responsible AI use.
- AI-Powered Personal Finance: AI will play an increasingly significant role in personal finance, helping individuals make smarter investment decisions and manage their wealth.
Conclusion
In conclusion, the role of AI in hedge funds and private equity is reshaping the financial industry. AI’s ability to process vast datasets, make data-driven decisions, and enhance investment strategies is redefining how wealth is managed and grown. While AI offers substantial benefits, it also poses ethical and practical challenges that must be addressed to ensure responsible and transparent use.
As AI continues to advance, the finance industry will see more innovative applications, improved risk management, and enhanced investment opportunities. The key to success lies in finding the right balance between AI-driven decision-making and the human expertise that guides it. By staying informed about these developments, investors and financial professionals can adapt to the changing landscape and harness the power of AI to achieve their financial goals.
Additional Resources
For those interested in delving deeper into this topic, we recommend exploring the following resources:
- [Book] “The AI Advantage” by Thomas H. Davenport
- [Online Platform] “AI in Finance” by The CFA Institute
- [Research Paper] “Machine Learning in Asset Management” by Marcos López de Prado
Remember that AI in finance is a dynamic field, and staying informed and adaptable is crucial to harness its full potential.